文章目录
- 补:交叉验证+参数调优(网格搜索)
- 1.opencv的核心技术
- 2.opencv的c++开发
- 3.opencv的python开发
-
- 3.1 特征点检测的使用
- 3.2 特征描述与特征匹配
- 3.3 人脸识别
- 4.人脸识别+交叉验证
补:交叉验证+参数调优(网格搜索)
import numpy as np
from sklearn import datasets
from sklearn.model_selection import train_test_split,GridSearchCV
from sklearn.svm import SVC
data,target=datasets.load_iris(return_X_y=True)
print(data.shape)
data_train,data_test,target_train,target_test=train_test_split(data,target,test_size=0.2)
print(data_train.shape)
dic_p={
'C':[1,10,100,10000],'gamma':[0.01,0.1,1],
}
classifier=GridSearchCV(SVC(kernel='rbf'),dic_p)
classifier.fit(data_train,target_train)
pre=classifier.predict(data_test)
print((pre==target_test).sum())
(150, 4)
(120, 4)
30
1.opencv的核心技术
2.opencv的c++开发
3.opencv的python开发
3.1 特征点检测的使用
import cv2
import matplotlib.pyplot as plt
img_src=cv2.imread('timg.jpg')
img_src=cv2.cvtColor(img_src,cv2.COLOR_BGR2RGB)
orb=cv2.ORB_create(10000)
keypoints=orb.detect(img_src)
img_out=cv2.drawKeypoints(img_src,keypoints,None,(255,0,0))
plt.imshow(img_out)
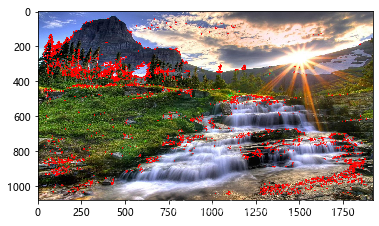
3.2 特征描述与特征匹配
import cv2
import matplotlib.pyplot as plt
img1=cv2.imread('timg.jpg')
img2=cv2.imread('timg2.jpg')
orb=cv2.ORB_create(500)
kp1=orb.detect(img1)
kp2=orb.detect(img2)kp1,desc1=orb.compute(img1,kp1)
kp2,desc2=orb.compute(img2,kp2)
bf=cv2.BFMatcher(cv2.NORM_HAMMING,crossCheck=True)
matches=bf.match(desc1,desc2)
img_out=cv2.drawMatches(img1,kp1,img2,kp2,matches,None)
plt.imshow(img_out)
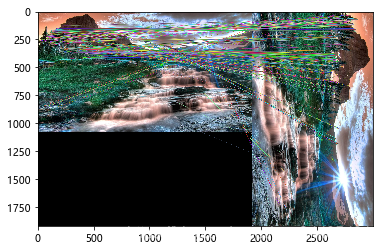
3.3 人脸识别
import numpy as np
import cv2
from cv2 import face
ONE_PERSON_FACE_NUM = 10
PERSON_NUM = 40
SAMPLE_NUM = ONE_PERSON_FACE_NUM * PERSON_NUM
IMG_W = 92
IMG_H = 112
data_faces = np.zeros(shape=(SAMPLE_NUM, IMG_W * IMG_H), dtype=np.int32)
label_faces = np.zeros(shape=(SAMPLE_NUM, 1), dtype=np.int32)
idx = 0
for i in range(1, PERSON_NUM + 1): for j in range(1, ONE_PERSON_FACE_NUM + 1):path_ = "./att_faces/s{i}/{j}.pgm".format(i=i,j=j)img_ = cv2.imread(path_)gray_ = cv2.cvtColor(img_, cv2.COLOR_BGR2GRAY)data_faces[idx, :] = gray_.reshape(IMG_W * IMG_H)label_faces[idx, :] = iidx += 1
model=face.FisherFaceRecognizer_create()
model.train(data_faces,label_faces)
label,conf=model.predict(data_faces[0])
print(label)
print(conf)
1
0.0
4.人脸识别+交叉验证
import numpy as np
#机器视觉方面使用opencv
import cv2
from cv2 import face
from sklearn.model_selection import train_test_split,GridSearchCV
from sklearn.metrics import classification_report# 接下来读取图像到矩阵,一共有四百张图
# 定义常量#每个人有十张自己的图像
ONE_PERSON_FACE_NUM = 10
#一共有40个人
PERSON_NUM = 40
#图像数量自然是40*10
SAMPLE_NUM = ONE_PERSON_FACE_NUM * PERSON_NUM
#每张图像高112,宽92
IMG_W = 92
IMG_H = 112#定义存放图像信心的矩阵
#因为有400张图,因此行是SAMPLE_NUM,列是每张图的信息,最后效果是一行代表一张图
data_faces = np.zeros(shape=(SAMPLE_NUM, IMG_W * IMG_H), dtype=np.int32)
#一行代表一个图片的类别,因此只需要一列
label_faces = np.zeros(shape=(SAMPLE_NUM, 1), dtype=np.int32)#把图片信息复制到矩阵中
idx = 0 # 数据集的位置,即在哪个文件夹
for i in range(1, PERSON_NUM + 1): # 40个人的目录,此处是使角标从1到PERSON_NUM,因为range不包含PERSON_NUM,所以得+1for j in range(1, ONE_PERSON_FACE_NUM + 1):path_ = "./att_faces/s{i}/{j}.pgm".format(i=i,j=j)img_ = cv2.imread(path_)#因为读取的是三通道图像,为了大大减少计算量,转化为灰度图像(因为做图像识别,颜色没有太大价值,所以可转灰度)gray_ = cv2.cvtColor(img_, cv2.COLOR_BGR2GRAY)data_faces[idx, :] = gray_.reshape(IMG_W * IMG_H)label_faces[idx, :] = iidx += 1#处理数据,将数据分离成训练数据和测试数据
#test_size=0.2,测试集是原数据规模的0.2
data_faces_train,data_faces_test,label_faces_train,label_faces_test=train_test_split(data_faces,label_faces,test_size=0.2)
#因为一共400张图,所以输出的test的行是80行,即代表有80个测试数据
print(data_faces_test.shape)#人脸分类算法
#model=face.EigenFaceRecognizer_create()#这个是基于特征脸的算法
model=face.FisherFaceRecognizer_create()#基于fisher判别式的算法
#使用训练数据训练模型
model.train(data_faces_train,label_faces_train)#用测试集第一张图看看模型预测效果
# label,conf=model.predict(data_faces_test[0]) #label是图的类型即标签,conf是执行度,执行度越小越好
# print(label_faces_test[79])
# print(label)
# print(conf)#pre_labels存放所有测试数据的测试标签
pre_labels=[]
for i in range(data_faces_test.shape[0]):val,conf=model.predict(data_faces_test[i])pre_labels.append(val)#用real_labels保存label_faces_test的一维数据形式
real_labels=label_faces_test.reshape(1, -1)
print("pre_labels:",pre_labels)
print("real_labels:",real_labels)
report = classification_report(pre_labels, label_faces_test[:,0])
print(report)
(80, 10304)
pre_labels: [39, 15, 28, 25, 9, 35, 10, 4, 11, 20, 37, 26, 34, 6, 16, 37, 22, 12, 12, 39, 10, 23, 15, 2, 3, 29, 13, 24, 11, 31, 36, 39, 5, 26, 7, 8, 37, 36, 33, 35, 9, 16, 23, 38, 31, 9, 30, 20, 6, 19, 24, 5, 17, 24, 21, 8, 23, 9, 18, 7, 4, 9, 26, 27, 40, 31, 27, 19, 20, 12, 40, 15, 17, 36, 28, 36, 24, 40, 35, 39]
real_labels: [[39 15 28 25 9 35 10 4 11 33 37 26 34 6 16 37 22 12 12 39 10 23 15 23 29 13 24 11 31 36 39 5 26 7 19 37 36 33 35 9 16 23 38 31 9 33 206 19 24 5 17 24 21 8 23 9 18 7 4 9 26 27 40 31 27 19 20 12 35 1517 36 28 36 24 40 35 39]]precision recall f1-score support2 1.00 1.00 1.00 13 1.00 1.00 1.00 14 1.00 1.00 1.00 25 1.00 1.00 1.00 26 1.00 1.00 1.00 27 1.00 1.00 1.00 28 1.00 0.50 0.67 29 1.00 1.00 1.00 510 1.00 1.00 1.00 211 1.00 1.00 1.00 212 1.00 1.00 1.00 313 1.00 1.00 1.00 115 1.00 1.00 1.00 316 1.00 1.00 1.00 217 1.00 1.00 1.00 218 1.00 1.00 1.00 119 0.67 1.00 0.80 220 1.00 0.67 0.80 321 1.00 1.00 1.00 122 1.00 1.00 1.00 123 1.00 1.00 1.00 324 1.00 1.00 1.00 425 1.00 1.00 1.00 126 1.00 1.00 1.00 327 1.00 1.00 1.00 228 1.00 1.00 1.00 229 1.00 1.00 1.00 130 0.00 0.00 0.00 131 1.00 1.00 1.00 333 0.33 1.00 0.50 134 1.00 1.00 1.00 135 0.75 1.00 0.86 336 1.00 1.00 1.00 437 1.00 1.00 1.00 338 1.00 1.00 1.00 139 1.00 1.00 1.00 440 1.00 0.67 0.80 3accuracy 0.95 80macro avg 0.94 0.94 0.93 80
weighted avg 0.96 0.95 0.95 80