Airbnb是AirBed and Breakfast ( “Air-b-n-b” )的缩写,中文名称之为:空中食宿,是一家联系旅游人士和家有空房出租的服务型网站,可以为用户提供各式各样的住宿信息。
本文针对kaggle上爱彼迎在新加坡的一份数据进行探索分析。原notebook学习地址:https://www.kaggle.com/bavalpreet26/singapore-airbnb/notebook
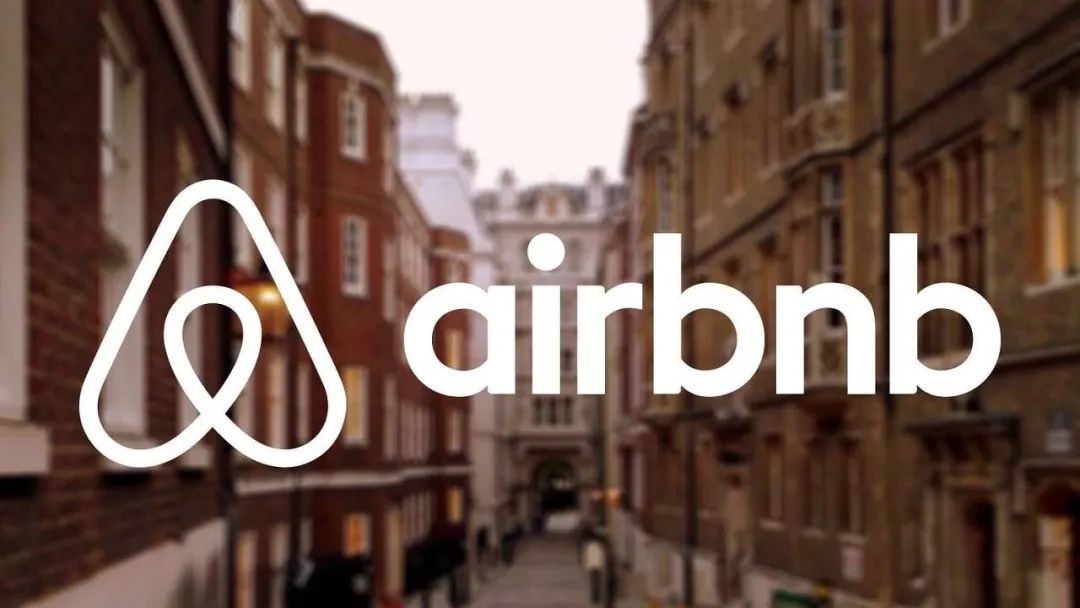
爱彼迎将全球的租房数据进行了收集,并且放在了自己的官网上供参考,官方数据地址:http://insideairbnb.com/get-the-data.html
上面很多城市的数据,国内的有北京、上海等,都是免费可下载的,感兴趣的朋友可以玩转这些数据。
本文选择的是花园城市-狮城新加坡,是个出国旅游的好去处!

本文相关数据集和代码大家可以直接在Kaggle官网下载。
导入库
导入数据分析需要的库:
import pandas as pd
import numpy as np# 二维图形
import matplotlib
import matplotlib.pyplot as plt
import seaborn as sns
import geopandas as gpd
plt.style.use('fivethirtyeight')
%matplotlib inline# 动态图
import plotly as plotly
import plotly.express as px
import plotly.graph_objects as go
from plotly.offline import init_notebook_mode, iplot, plot
init_notebook_mode(connected=True)# 地图制作
import folium
import folium.plugins# NLP:词云图
import wordcloud
from wordcloud import WordCloud, STOPWORDS, ImageColorGenerator# 机器学习建模相关
import sklearn
from sklearn import preprocessing
from sklearn import metrics
from sklearn.metrics import r2_score, mean_absolute_error
from sklearn.preprocessing import LabelEncoder,OneHotEncoder
from sklearn.model_selection import train_test_split
from sklearn.linear_model import LinearRegression,LogisticRegression
from sklearn.ensemble import RandomForestRegressor, GradientBoostingRegressor# 忽略告警
import warnings
warnings.filterwarnings("ignore")
数据基本信息
导入我们获取到的数据:
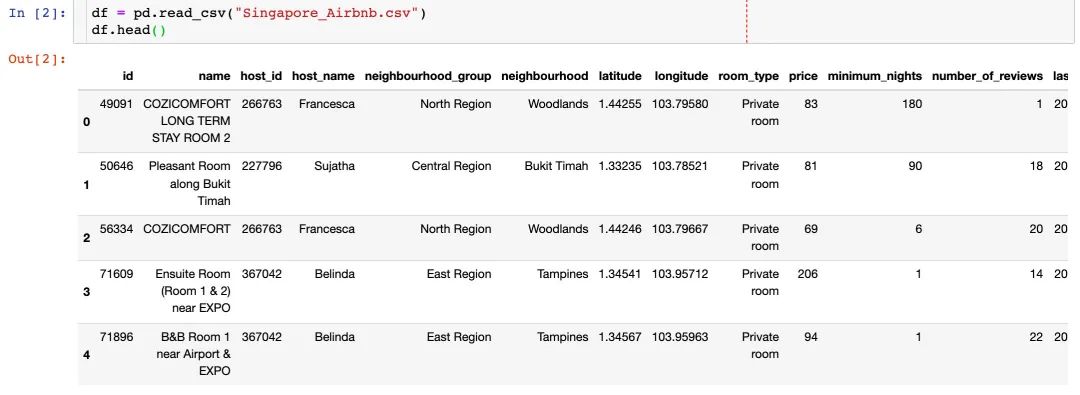
查看数据的基本信息:形状shape、字段、缺失值等
# 数据形状
df.shape(7907, 16)# 字段信息
columns = df.columns
columnsIndex(['id', 'name', 'host_id', 'host_name', 'neighbourhood_group','neighbourhood', 'latitude', 'longitude', 'room_type', 'price','minimum_nights', 'number_of_reviews', 'last_review','reviews_per_month', 'calculated_host_listings_count','availability_365'],dtype='object')
具体解释下每个字段的中文含义为:
id:记录ID
name:房屋名字
host_id:房东id
host_name:房东名字
neighbourhood:区域
latitude:纬度
longitude:经度
room_type:房间类型
price:价格
minimum_nights:预订最低天数
number_of_reviews:评论数量
last_reviews:最近一次评论时间
reviews_per_month:评论数/月
calculated_host_listings_count:房东拥有的可出租房屋数量
availability_365:房屋一年内可租天数
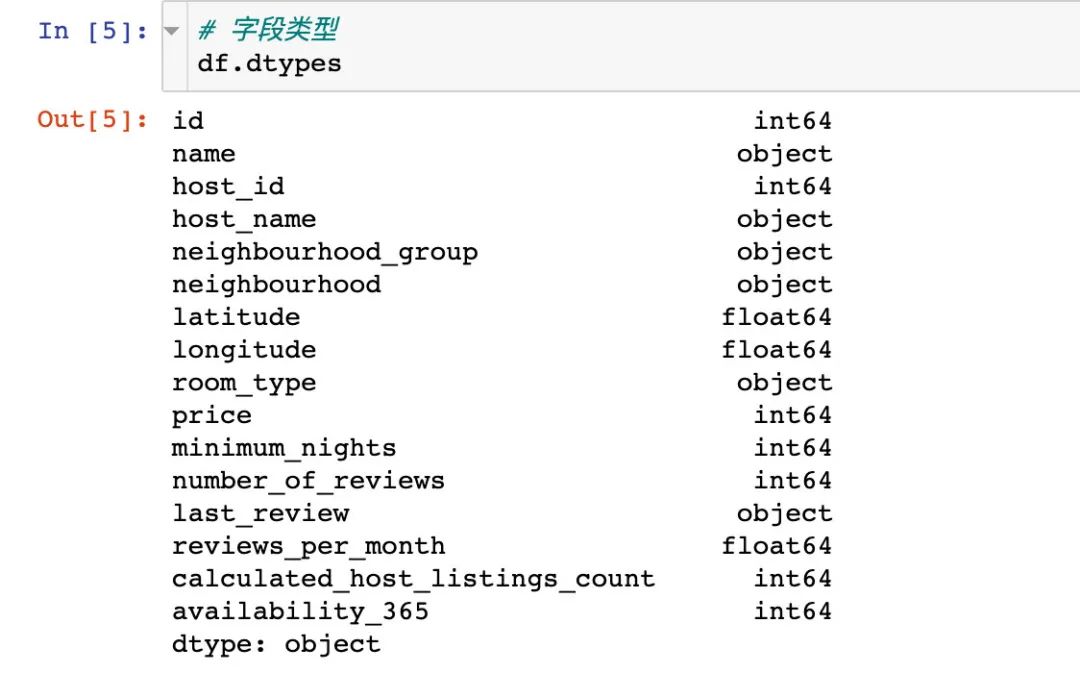
通过DataFrame的info属性我们能够查看数据的多个信息:
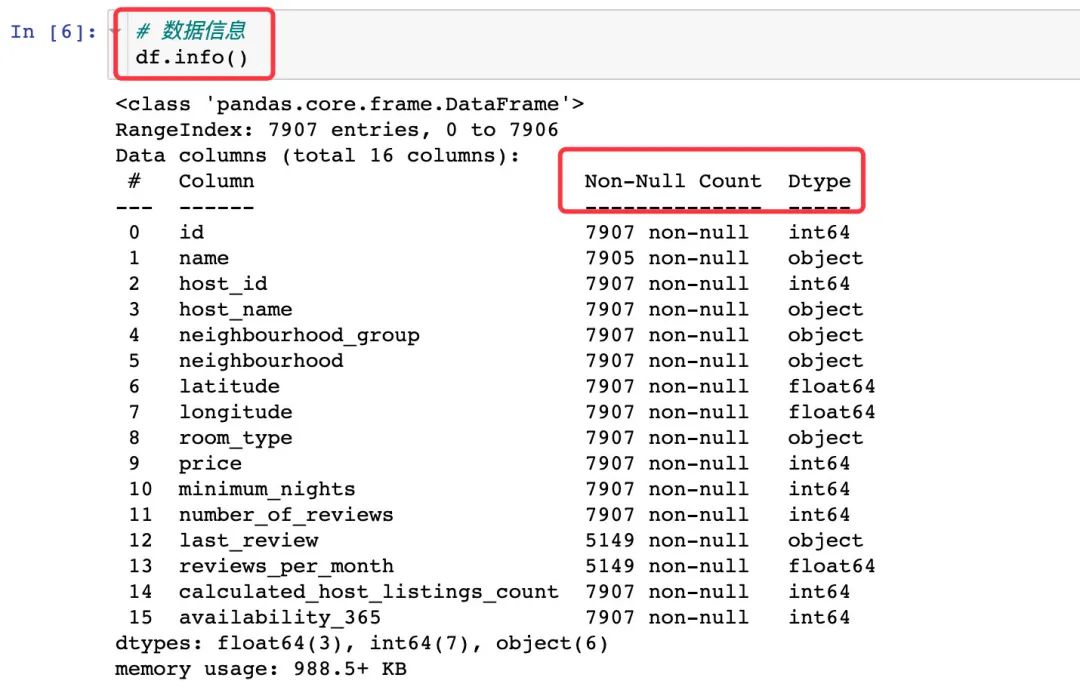
具体的缺失值情况:
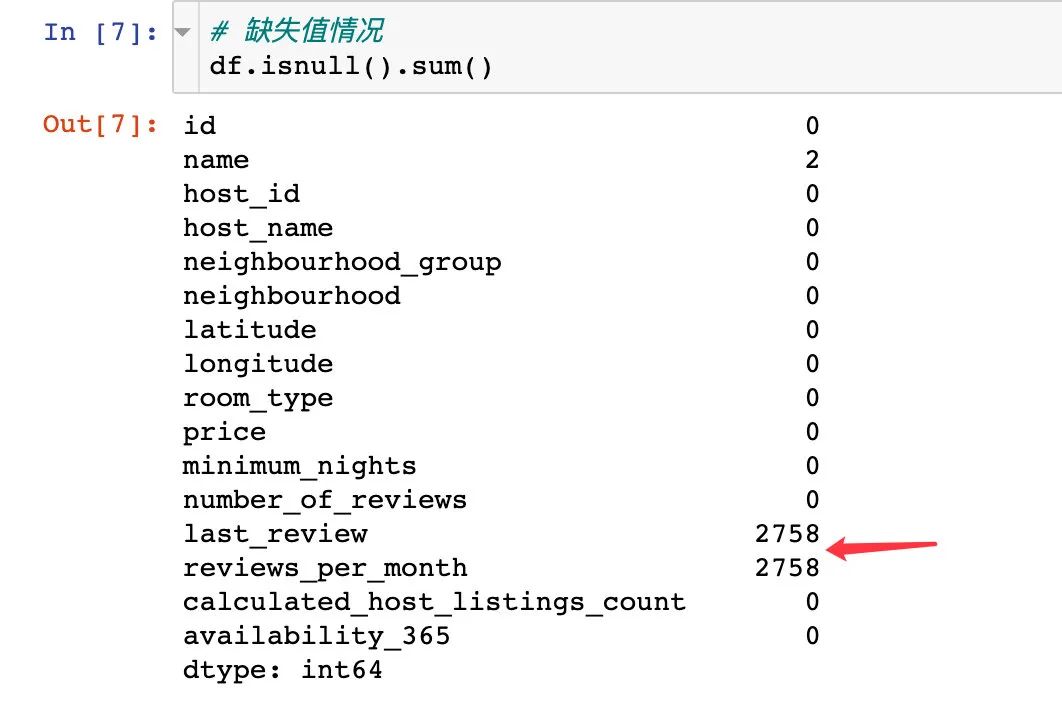
缺失值处理
1、先查看字段的缺失值分布情况:从下面的图形中看出来也是last_review和reviews_per_month字段存在缺失值
sns.set(rc={'figure.figsize':(19.7, 8.27)})
sns.heatmap(df.isnull(),yticklabels=False,cbar=False,cmap='viridis'
)plt.show()
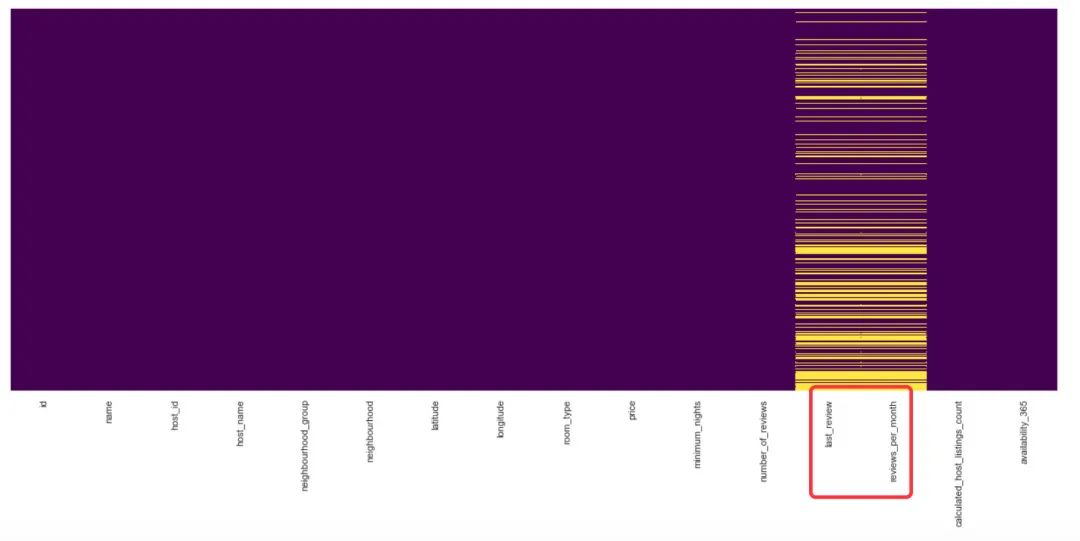
2、缺失值的字段(上面的两个)和name字段的两行记录直接删除
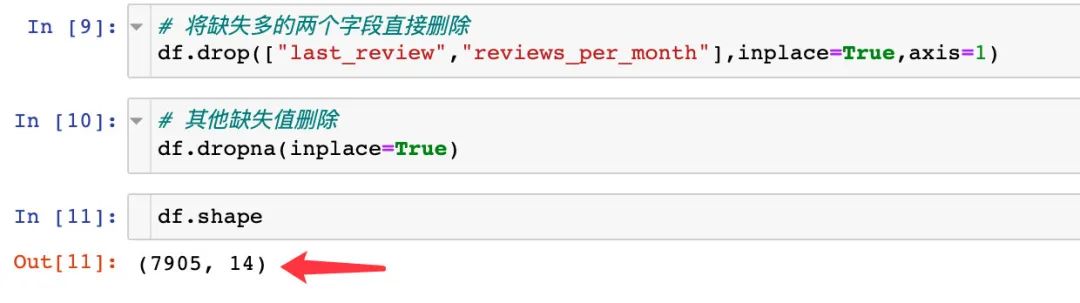
最终的数据变成了7905行和14个字段。原始数据是7907行,16个字段属性
数据EDA
EDA全称是:Exploratory Data Analysis,主要是为了探索数据的分布情况
价格price
整体的话,价格还是在1000以下
sns.distplot(df["price"]) # 直方图
plt.show()
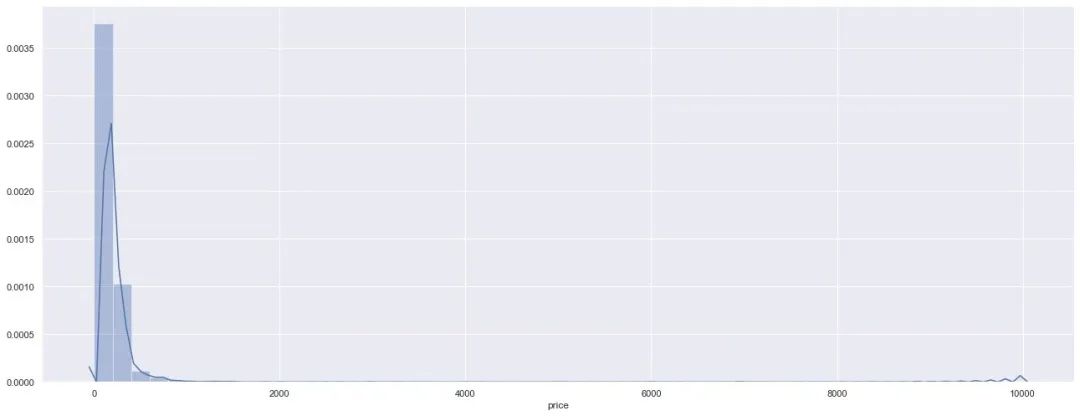
下面我们看看价格和最低预订天数的关系:
sns.scatterplot(x="price",y="minimum_nights", # 每夜最少data=df)plt.show()
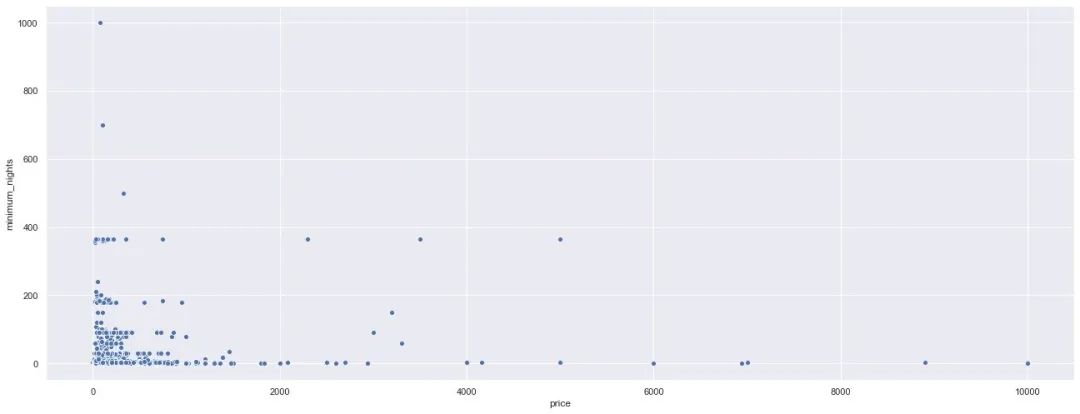
通过价格的散点图,也能够观察到主要的价格还是分布在最低预订天数在200以下的房源中
区域
查看房屋的区域(地理为)分布:更多的房子位于Central Region位置。
sns.countplot(df["neighbourhood_group"])
plt.show()
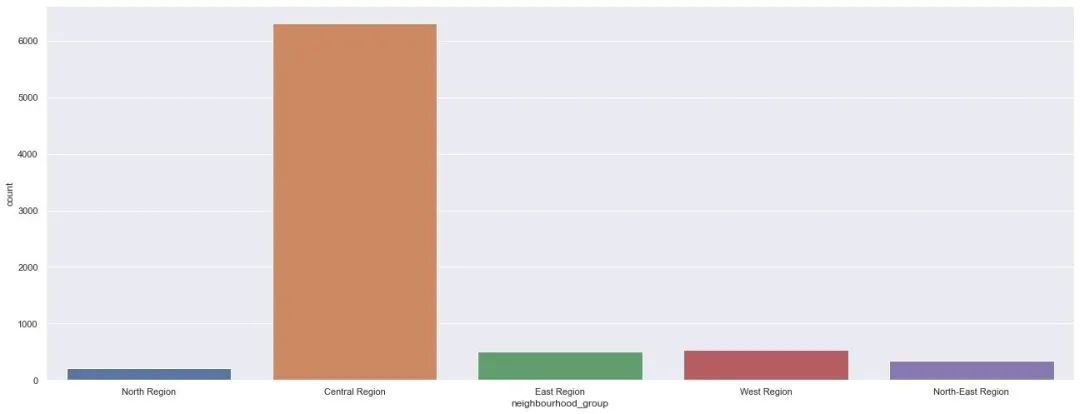
上面是从房源的数量上对比每个区域,下面是对比不同
df1 = df[df.price < 250] # 小于250房子较多
plt.figure(figsize=(10,6))sns.boxplot(x = 'neighbourhood_group',y = 'price',data=df1)plt.title("neighbourhood_group < 250")plt.show()
从箱型图中观察到:Central Region区域的房子
房价分布更为宽广
房价的均值也高于其他位置
价格分布没有比较其他的值,较为合理
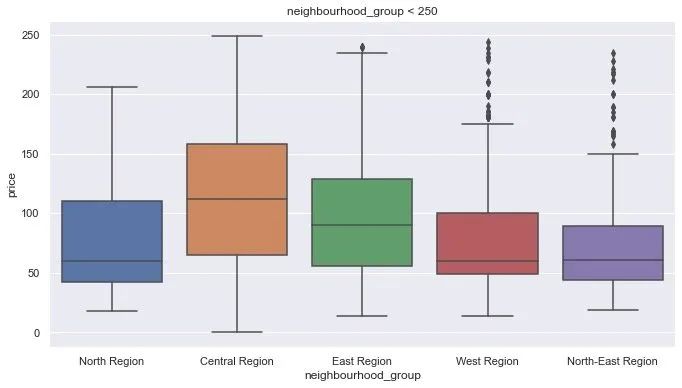
上面是从房子的区域来比较,下面可以找找它们的具体经纬度:
plt.figure(figsize=(12,8))sns.scatterplot(df.longitude,df.latitude,hue=df.neighbourhood_group)plt.show()
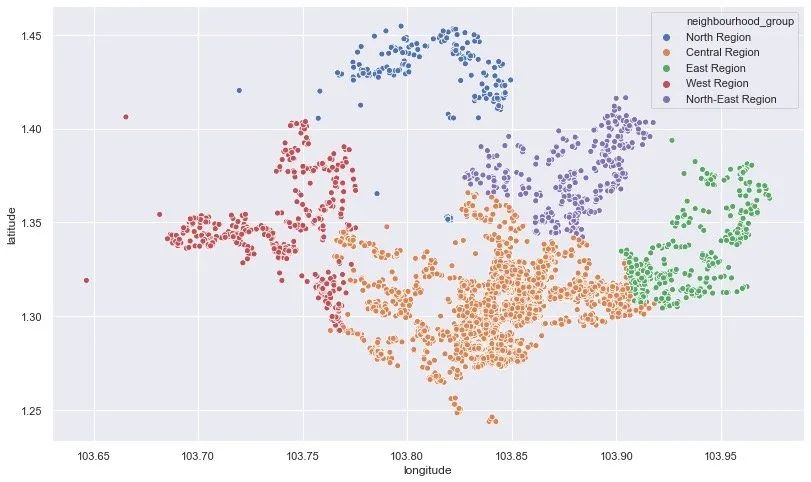
房源分布热力图
为了绘制地理位置的热力图,可以学下下这个库:folium
import folium
from folium.plugins import HeatMapm = folium.Map([1.44255,103.79580],zoom_start=11)HeatMap(df[['latitude','longitude']].dropna(),radius=10,gradient={0.2:'blue',0.4:'purple',0.6:'orange',1.0:'red'}).add_to(m)
display(m)
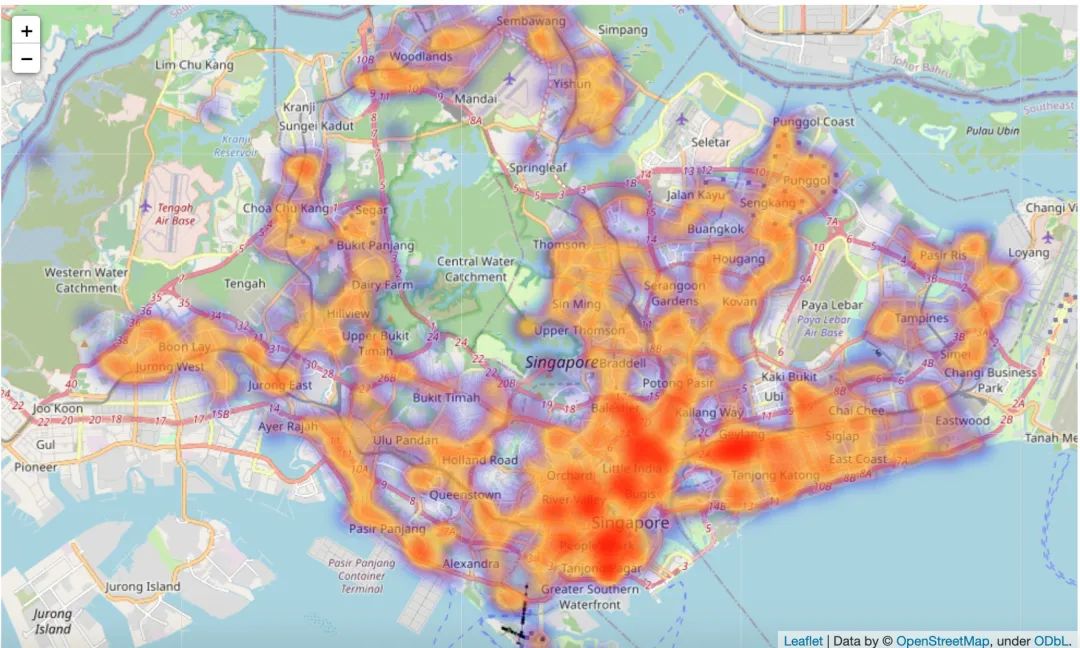
房间类型room_type
不同房间类型的占比
统计3种不同房间类型的总数和对应的百分比:
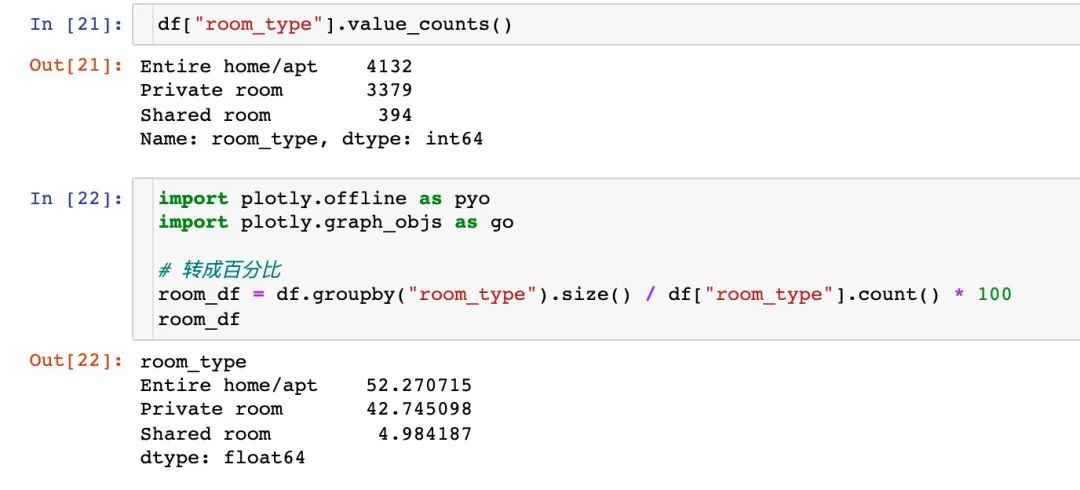
对这3种类型的占比进行可视化对比:
labels = room_df.index
values = room_df.valuesfig = go.Figure(data=[go.Pie(labels=labels,values=values,hole=0.5)])fig.show()
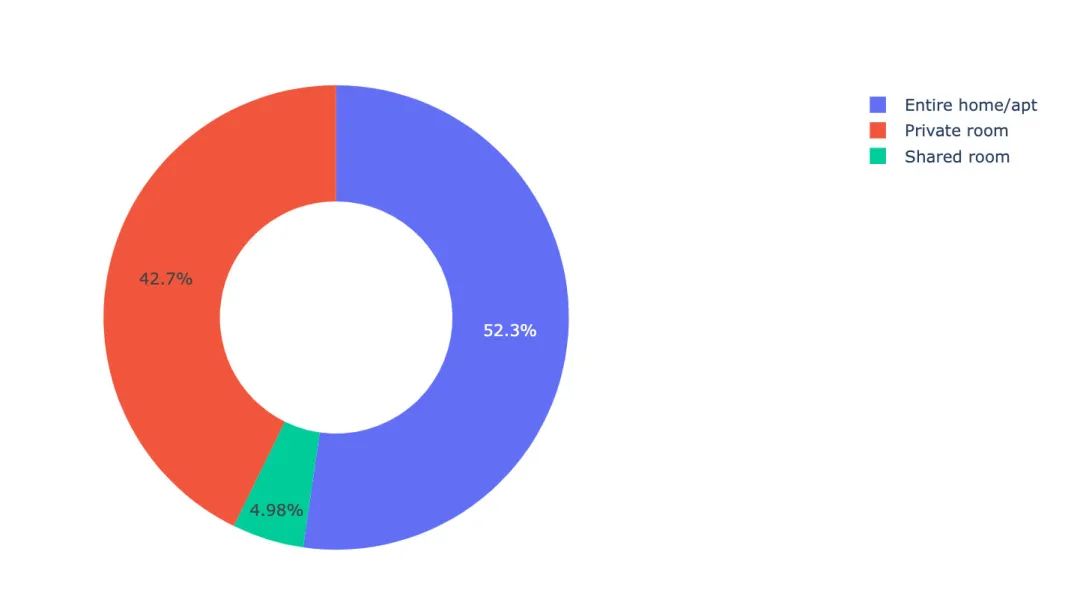
结论:整租或者公寓方式的房源占比最大,可能更受欢迎。
不同区域的房间类型
plt.figure(figsize=(12,6))sns.countplot(data = df,x="room_type",hue="neighbourhood_group"
)plt.title("room types occupied by the neighbourhood_group")
plt.show()
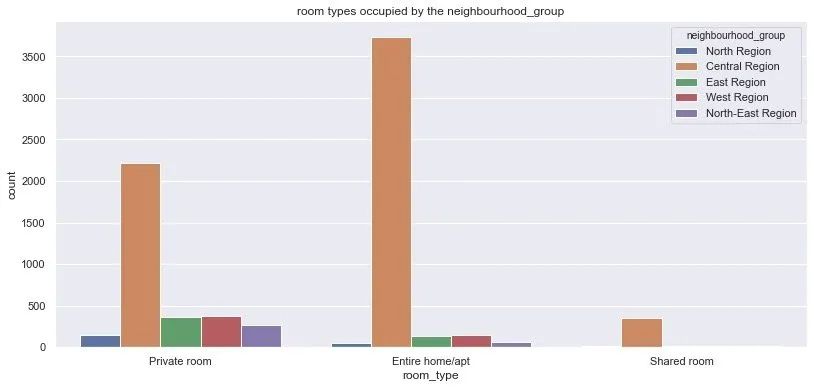
对比不同区域位置下的不同类型的房间,我们得到相同的结论:在不同的room_type下,Central Region位置的房间是最多的
个人增加部分:如何使用Plotly来绘制上面的分组状图?
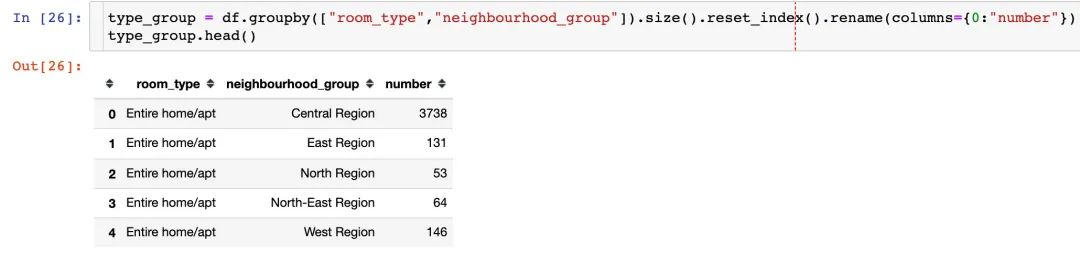
px.bar(type_group,x="room_type",y="number",color="neighbourhood_group",barmode="group")
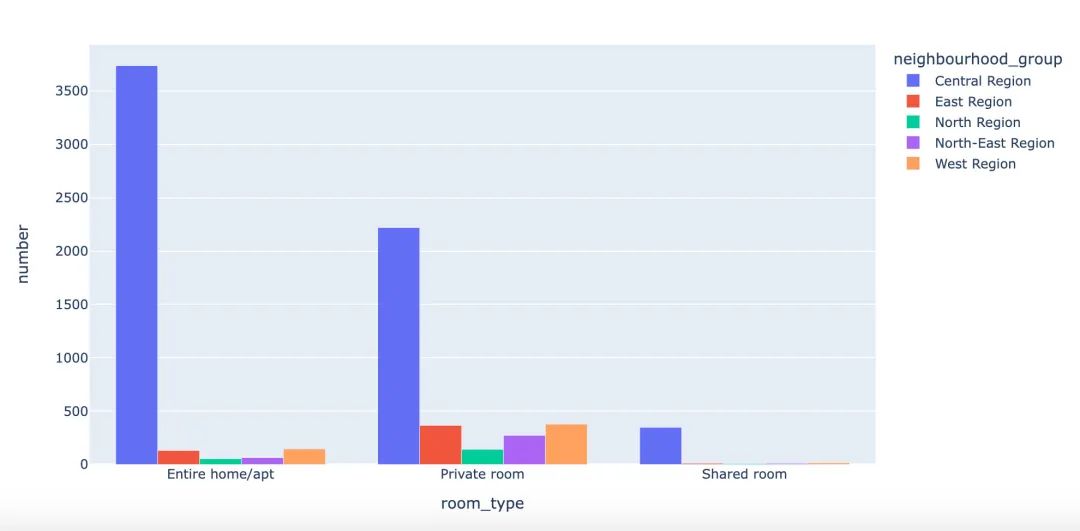
房间类型和价格关系
plt.figure(figsize=(12,6))sns.catplot(data=df,x="room_type",y="price")plt.show()
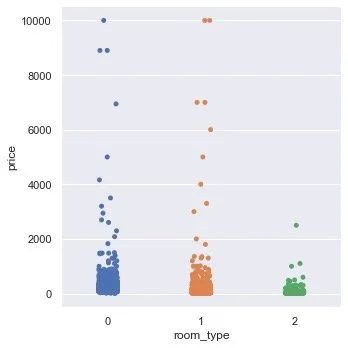
个人增加:使用Plotly绘制版本
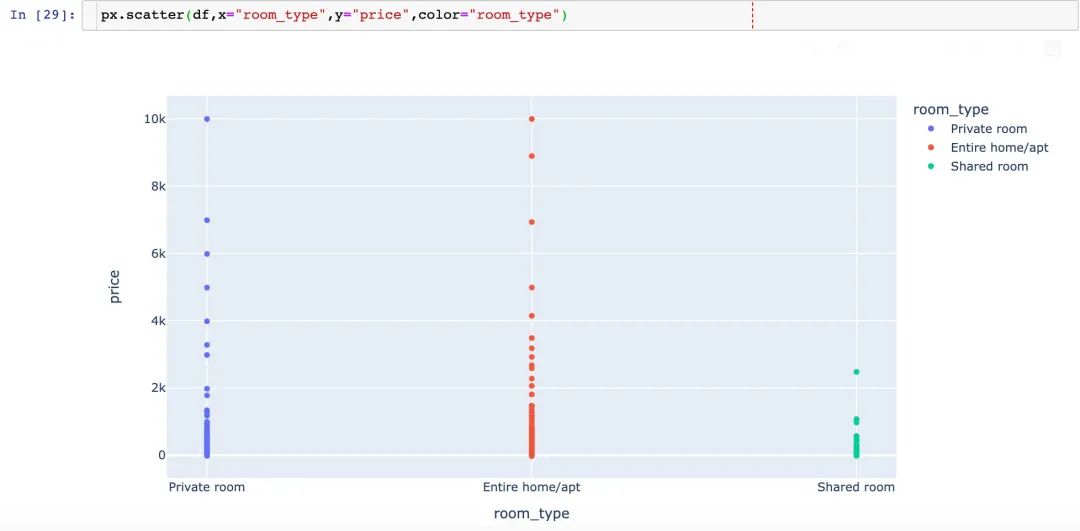
房间名称
整体词云图
绘制基于房间名称name的词云图:
from wordcloud import WordCloud, ImageColorGenerator
text = " ".join(str(each) for each in df.name)wordcloud = WordCloud(max_words=200,background_color="white").generate(text)plt.figure(figsize=(10,6))
plt.figure(figsize=(15,10))
plt.imshow(wordcloud, interpolation="Bilinear")plt.axis("off")
plt.show()
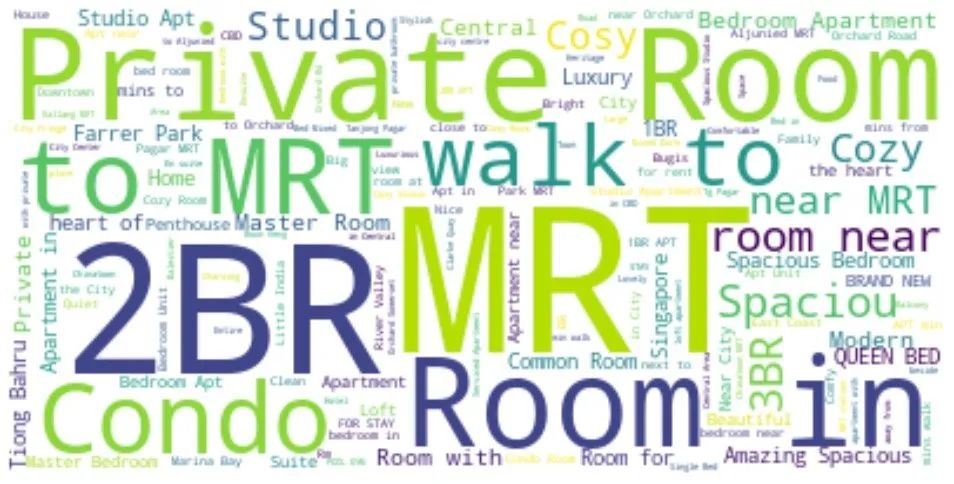
2BR:2 Bedroom Apartments,两室房
MRT:Mass Rapid Transit,新加坡的地铁;可能是靠近地铁的房子比较多
名字中的关键
将名字进行切割后其中的关键词:
# 将数据的名字全部装在列表names中
names = []
for name in df.name:names.append(name) def split_name(name):"""作用:切割每个名字"""spl = str(name).split()return splnames_count = []
for each in names: # 循环列表namesfor word in split_name(each): # 每个名字实行切割操作word = word.lower() # 统一变成小写names_count.append(word) # 每次切割的结果放入列表中# 计数库
from collections import Counter
result = Counter(names_count).most_common()
result[:5]

top_20 = result[0:20] # 前20个的高频词语top_20_words = pd.DataFrame(top_20, columns=["words","count"])
top_20_words
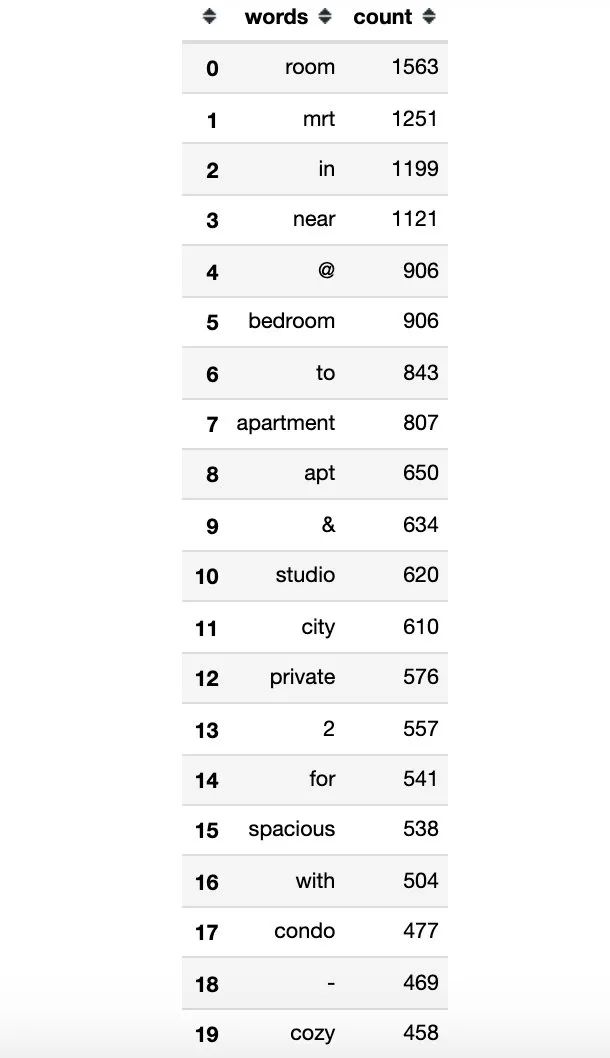
plt.figure(figsize=(10,6))fig = sns.barplot(data=top_20_words,x="words",y="count")
fig.set_title("Counts of the top 20 used words for listing names")
fig.set_ylabel("Count of words")
fig.set_xlabel("Words")
fig.set_xticklabels(fig.get_xticklabels(), rotation=80)
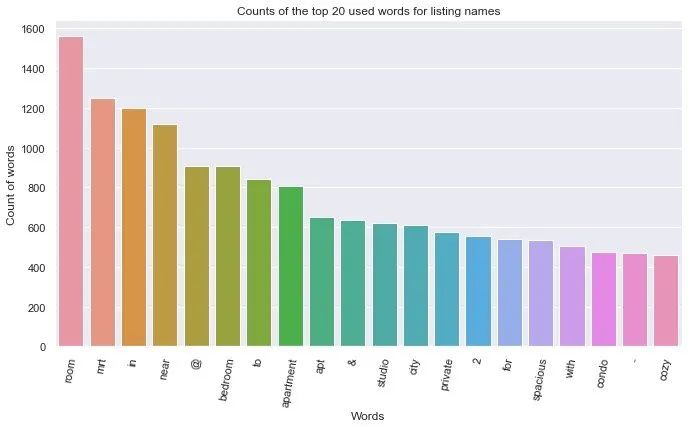
回访量统计
查看哪些房间的回访量较高:
df1 = df.sort_values(by="number_of_reviews",ascending=False).head(1000)df1.head()
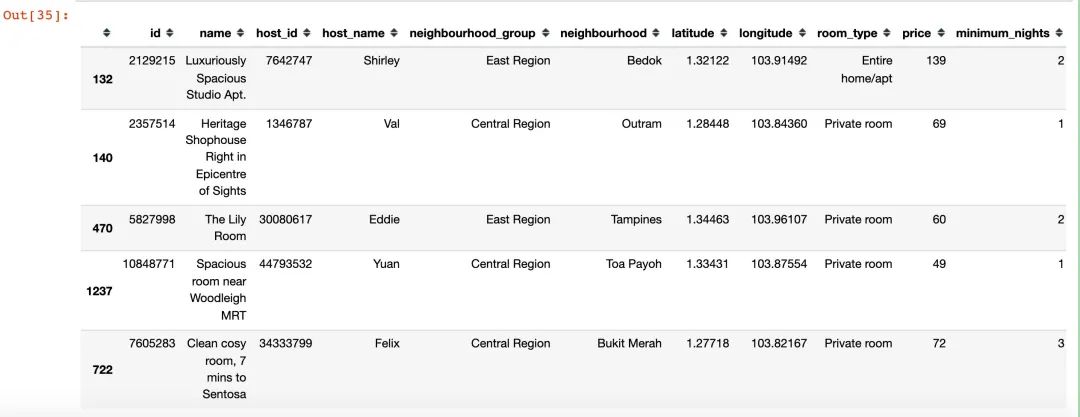
import folium
from folium.plugins import MarkerCluster
from folium import pluginsprint("Rooms with the most number of reviews")Long=103.91492
Lat=1.32122mapdf1 = folium.Map([Lat, Long], zoom_start=10)mapdf1_rooms_map = plugins.MarkerCluster().add_to(mapdf1)for lat, lon, label in zip(df1.latitude,df1.longitude,df1.name):folium.Marker(location=[lat, lon],icon=folium.Icon(icon="home"),popup=label).add_to(mapdf1_rooms_map)mapdf1.add_child(mapdf1_rooms_map)
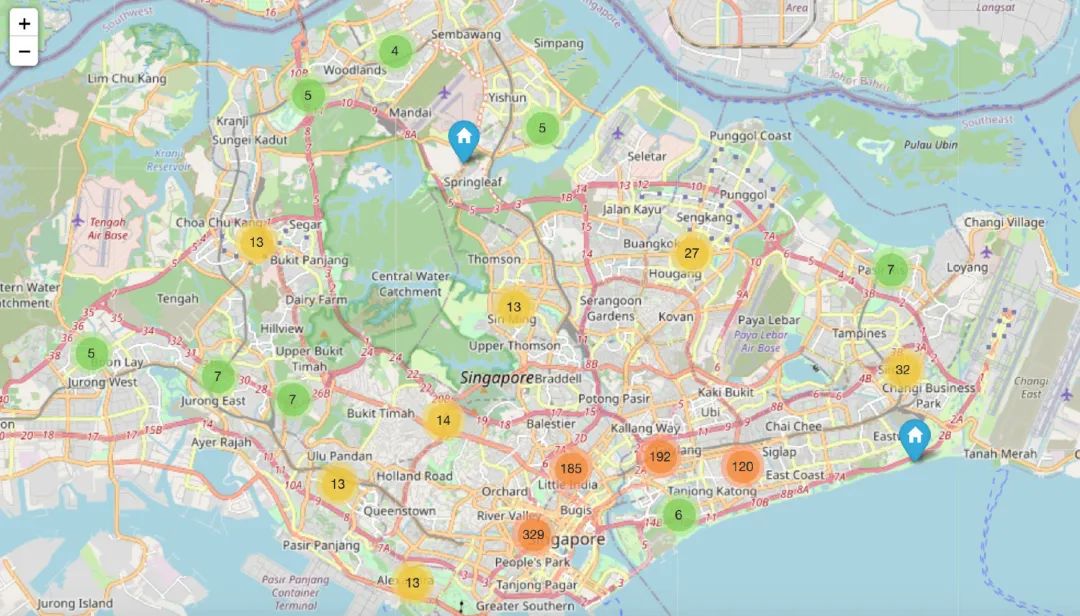
可租天数
在不同经纬度条件下,房子在一年中的可租天数对比:
plt.figure(figsize=(10,6))plt.scatter(df.longitude,df.latitude,c=df.availability_365,cmap="spring",edgecolors="black",linewidths=1,alpha=1)cbar=plt.colorbar()
cbar.set_label("availability_365")
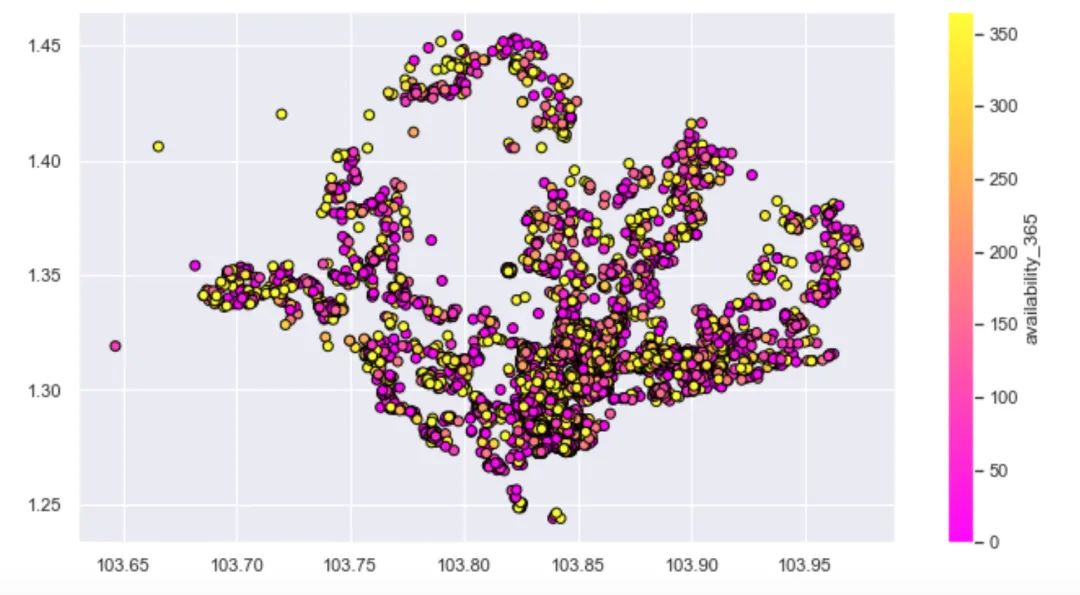
个人增加部分:使用Plotly如何绘制?
# plotly版本
px.scatter(df,x="longitude",y="latitude",color="availability_365")
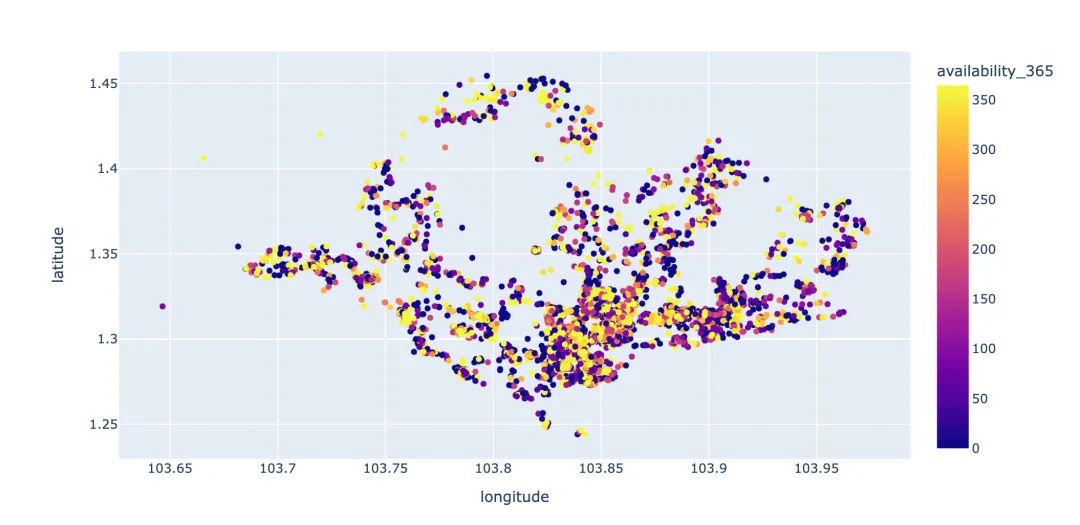
price小于500的房子的分布情况:
# price小于500的数据plt.figure(figsize=(10,6))
low_500 = df[df.price < 500]viz1 = low_500.plot(kind="scatter",x='longitude',y='latitude',label='availability_365',c='price',cmap=plt.get_cmap('jet'),colorbar=True,alpha=0.4)
viz1.legend()
plt.show()
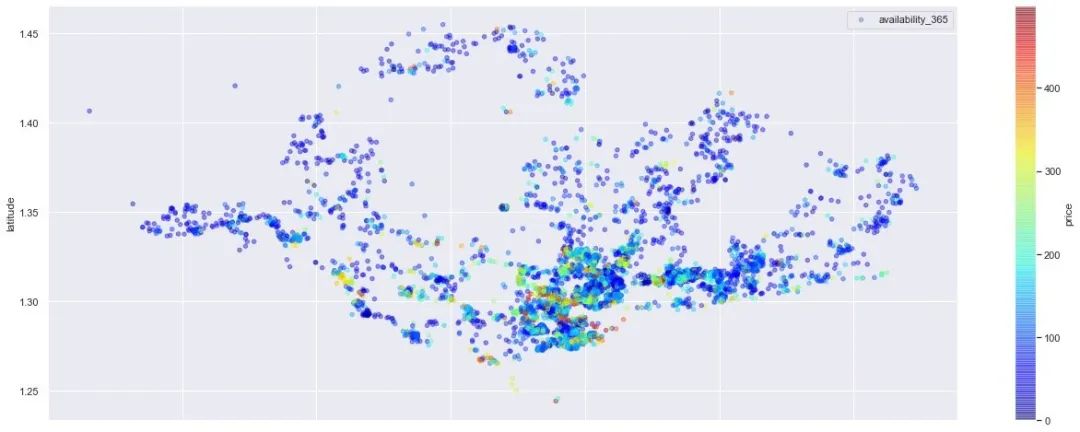
增加部分:更为简洁的Plotl8y版本
# plotly版本
px.scatter(low_500,x='longitude',y='latitude',color='price')
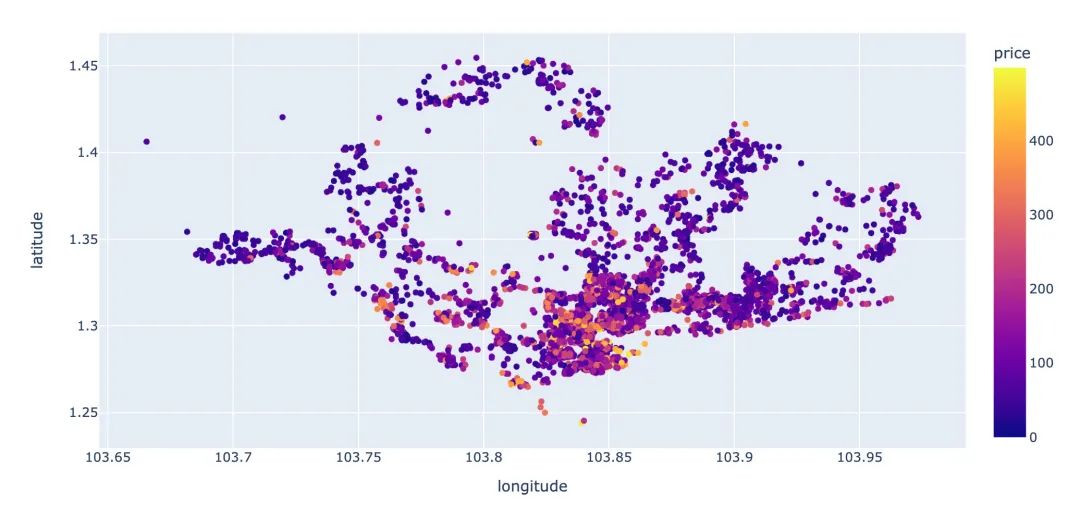
线性回归建模
预处理
基于线性回归的建模方案,先删除无效字段:
df.drop(["name","id","host_name"],inplace=True,axis=1)
编码类型的转化:
cols = ["neighbourhood_group","neighbourhood","room_type"]for col in cols:le = preprocessing.LabelEncoder()le.fit(df[col])df[col] = le.transform(df[col])df.head()

建模
# 模型实例化
lm = LinearRegression()# 数据集
X = df.drop("price",axis=1)
y = df["price"]# 训练集和测试集
X_train, X_test, y_train, y_test = train_test_split(X, y, test_size=0.2, random_state=101)lm.fit(X_train, y_train)
测试集验证
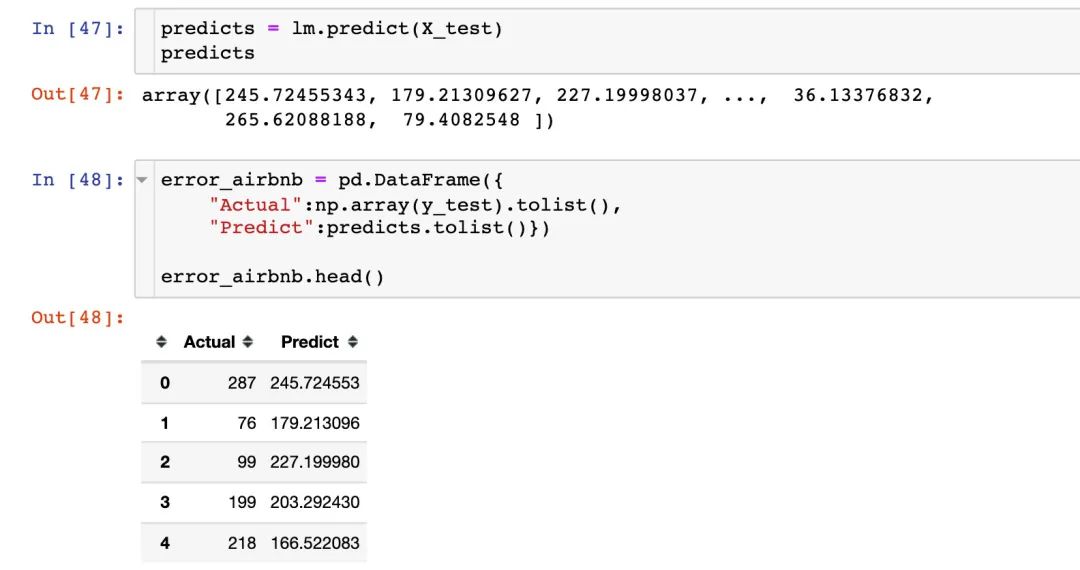
title=['Pred vs Actual']fig = go.Figure(data=[go.Bar(name='Predicted',x=error_airbnb.index,y=error_airbnb['Predict']),go.Bar(name='Actual',x=error_airbnb.index,y=error_airbnb['Actual'])
])fig.update_layout(barmode='group')
fig.show()
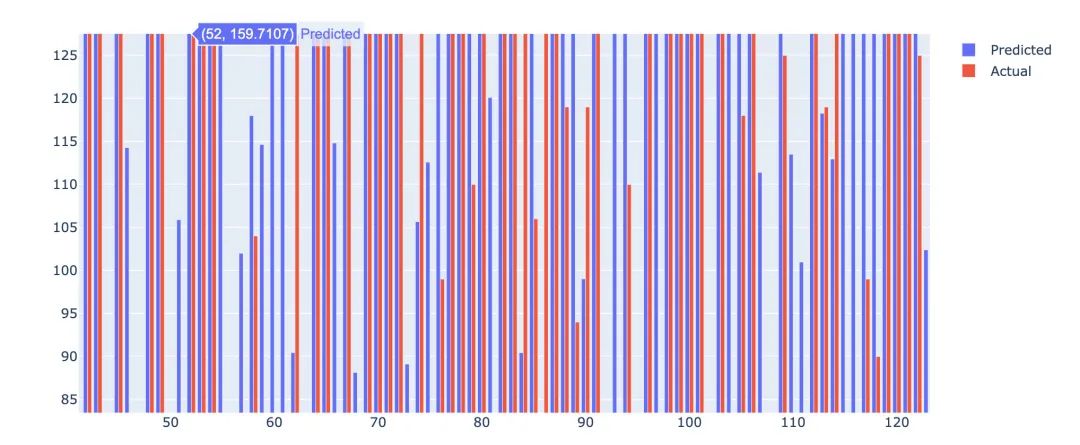
个人增加部分:我们对比预测值和真实值,做出二者的差值diff(增加字段)
error_airbnb["diff"] = error_airbnb["Predict"] - error_airbnb["Actual"]
px.box(error_airbnb,y="diff")
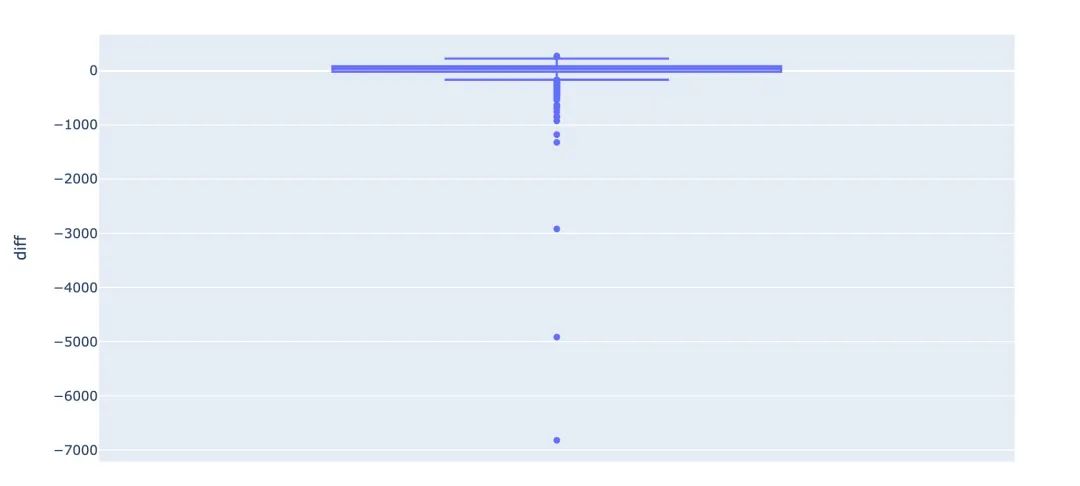
通过差异值diff的箱型图我们发现:真实值和预测值在有些数据中差别很大。
通过下面的descride属性也可以看到:有的居然相差了6820(绝对值),属于异常值的情况;四分之一的中位为-19,差值为19,整体上二者还是较为接近
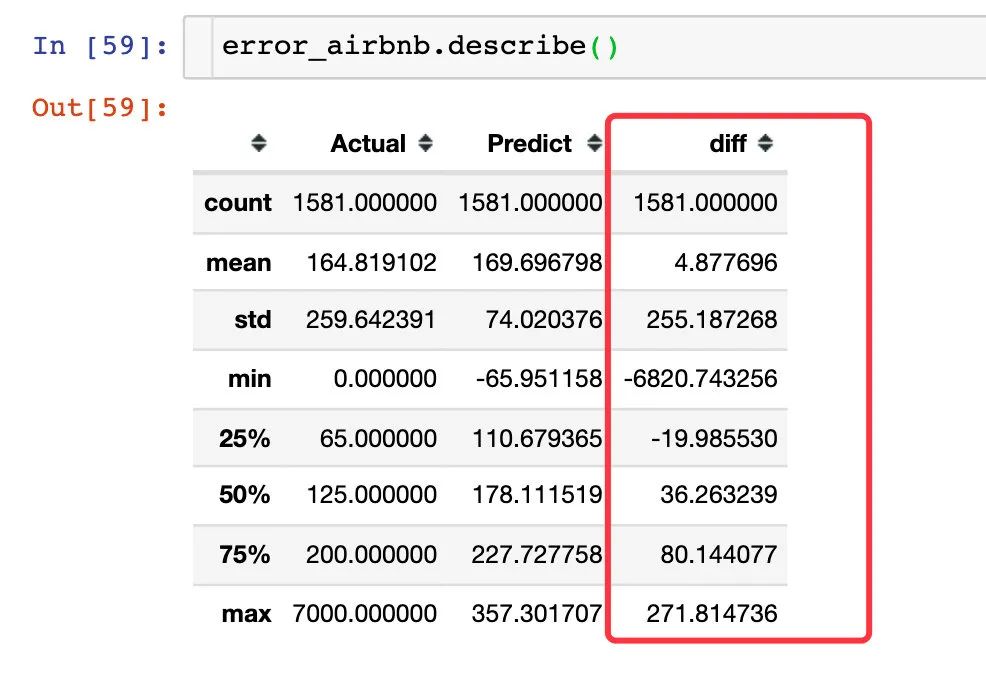
以上。
●总算是把用户流失分析讲清楚了!
●品牌知名度分析